
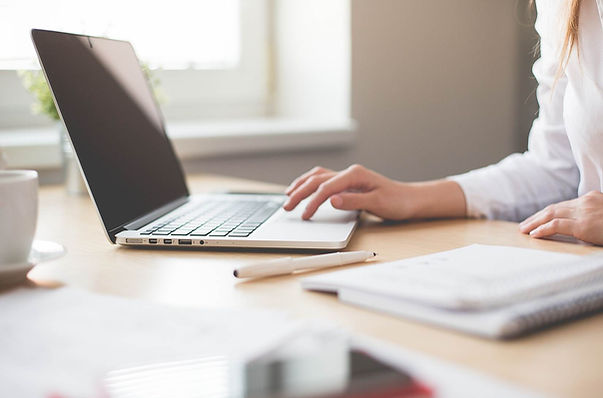
Education & Research
"Algorithm-drive applications are transforming educational practices and processes and places."
​
- Williamson, 2016
Educational data mining (EDM) is not new in education. According to Shahiri et al. (2015) “educational data mining is a process used to extract useful information and patterns from a huge educational database” (p. 415). EDM has been used to predict student’s ability to graduate on time (Porter & Balu, 2016) and identify slow learners (Kaur et al., 2015). Various types of algorithms have been analysed including Decision Tree, Neural Network, Naïve Bayes, K-Nearest Neighbor and Support Vector Machine. The most accurate predictor of student success has been Neural Network (93%) and Decision Tree (91%) according to Kaur et al. (2015). Predictive algorithms rely on variables to make predictions. Variables have been classified as internal, including assignments, marks, test scores and attendance, external variables including demographics such as gender, age, family background and parental occupation, and psychological variables (Shahiri et al, 2015).
"Predicting students performance is mostly useful to help the educators and learning improving their learning and teaching process." (p. 420)
​
- Shahiri et al, 2015
Shahiri et al. (2015) reviewed various types of predictive algorithms to find that Neural Network was able to predict student performance with 98% accuracy over a 13-year period. They also found that internal and external variables played more of a factor than psychological factors.
Although predictive algorithms are used in education, the prevalence of such is unknown. Algorithm-driven applications such as learning analytics and behaviour assessment is more prevalent with companies such as Pearson and ClassDojo (Williamson, 2016). Therefore, a gap in education is needed to further explore predictive algorithms and their ability to predict student success when re-taking a course.